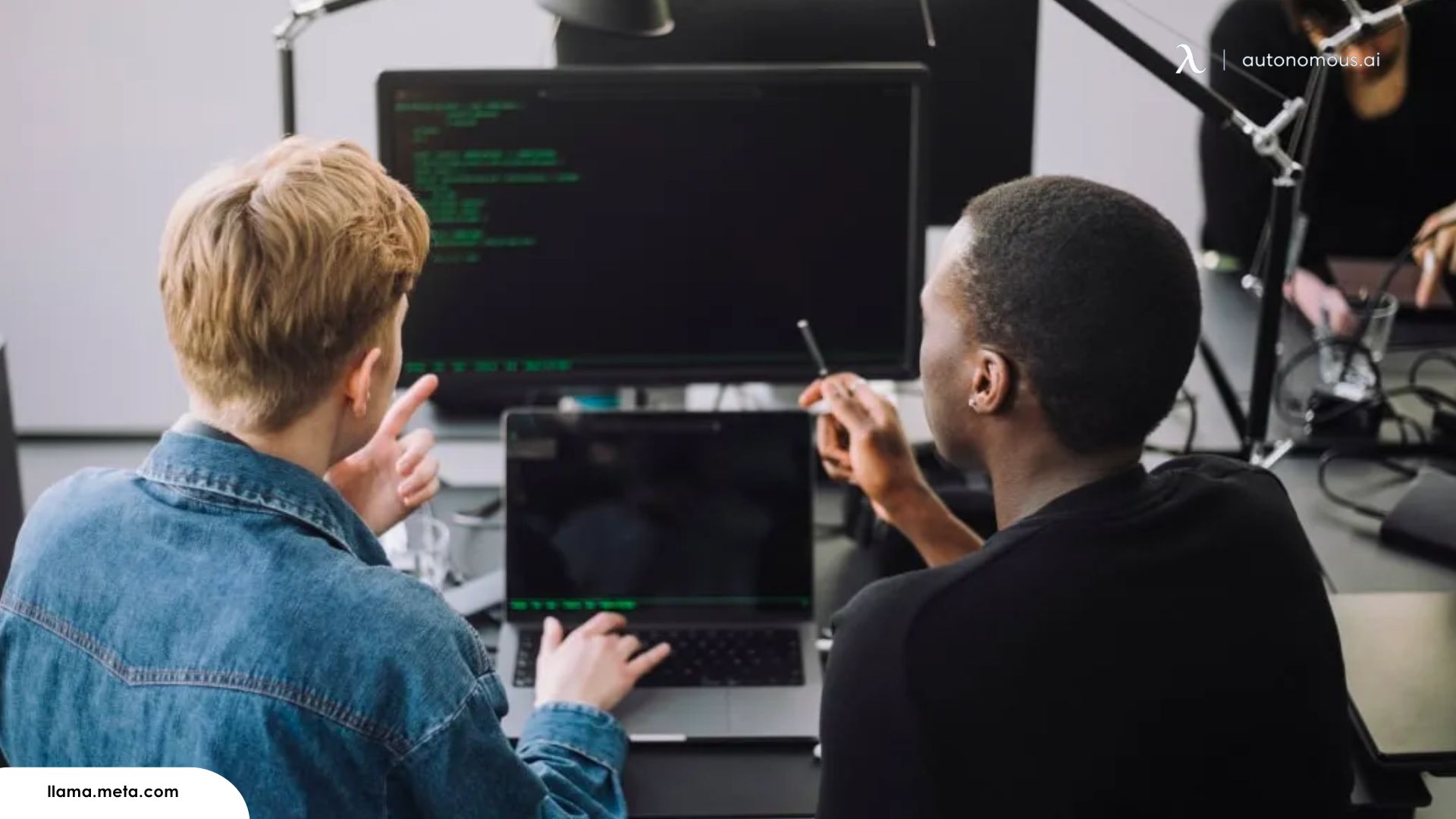
Vision-Language Models: Unlocking the Future of Multimodal AI
Table of Contents
Artificial intelligence is rapidly advancing, and one of the most transformative innovations is the rise of vision-language models (VLMs). These systems combine the power of computer vision and natural language processing (NLP) to process and understand both visual and textual data. By bridging the gap between these two modalities, VLMs are enabling groundbreaking applications across industries such as healthcare and e-commerce. This blog explores what vision-language models are, how they work, their benefits, applications, challenges, and the future potential of this cutting-edge AI technology.
1. What Are Vision-Language Models?
Vision-language models are a type of artificial intelligence designed to interpret and interact with both visual and textual inputs. These models enable machines to process images, videos, and text simultaneously, allowing them to perform tasks such as describing an image, answering questions about a video, or generating content based on visual cues. For businesses and individuals exploring advanced AI, many of the best AI tools now incorporate vision-language capabilities.
The foundation of VLMs lies in AI language models and multimodal learning, where data from different sources (e.g., images and text) is aligned and used to create a unified understanding. This capability has opened doors to applications that were previously limited to either vision or text-only systems.
- Enhanced Contextual Understanding: By processing text and visuals together, VLMs provide more nuanced and accurate interpretations of data.
- Broader Applications: VLMs are versatile and find applications across diverse fields, from healthcare to creative content generation.
- Improved Human-AI Interaction: Multimodal capabilities make AI systems more intuitive and responsive, enhancing user experiences.
1.1. How Do Vision-Language Models Work?
Vision-language models integrate advanced technologies to combine visual and textual data effectively:
- Data Alignment: VLMs align visual elements (e.g., objects in an image) with textual descriptions (e.g., captions) using paired datasets.
- Multimodal Embeddings: Using transformer-based architectures, VLMs encode visual and textual data into a shared representation space for seamless interaction.
- Pretraining on Large Datasets: These models are pretrained on datasets containing millions of image-text pairs, enabling them to generalize across various tasks.
- Cross-Modality Reasoning: VLMs analyze relationships between visual and textual data, allowing for tasks like answering questions about an image or generating a description for a video.
1.2. Applications of Vision-Language Models
Vision-language models are revolutionizing industries with their ability to process and understand multimodal inputs. Here are some key applications:
- Healthcare: VLMs assist in analyzing medical images (e.g., X-rays) and generating detailed natural language reports, improving diagnostic accuracy.
- E-commerce and Retail: Vision-language models enable visual search, personalized recommendations, and automatic tagging of product catalogs, enhancing customer experiences.
- Creative Industries: These models support content creators by generating descriptive captions, summarizing videos, and crafting visually informed stories.
- Accessibility: VLMs provide descriptive captions for images and videos, helping visually impaired individuals navigate digital and real-world environments.
- Autonomous Systems: Robots and drones use vision-language models to interpret surroundings and execute tasks requiring multimodal reasoning.
2. Popular Vision-Language Models
Several vision-language models are leading the charge in multimodal AI:
- CLIP (Contrastive Language-Image Pretraining): A model developed by OpenAI that aligns images and text for classification and retrieval tasks.
- DALL·E: Known for its text-to-image generation, DALL·E creates visuals based on textual descriptions. It is considered one of the best free AI image generators for transforming text into compelling images.
- Flamingo: Created by DeepMind, Flamingo specializes in tasks like visual question answering and video analysis, offering advanced reasoning capabilities for complex multimodal challenges.
- Llama 3.2 Vision: A widely used open-source large language model, Llama AI integrates visual and language reasoning capabilities. Its open-source nature makes it a versatile tool for developers working on diverse tasks in vision-language AI.
3. AnonAI: A Platform Leveraging Vision-Language Models
While not a vision-language model itself, AnonAI utilizes advanced VLMs like Llama 3.2 Vision to offer privacy-focused, multimodal solutions. AnonAI empowers users to integrate text and visuals seamlessly, whether for creative brainstorming, data analysis, or generating text-based insights from images. It also serves as a reliable private AI image generator for users looking to create visual content with enhanced privacy.
Its commitment to privacy and local data storage ensures secure, ethical use of these advanced technologies. As a trusted private AI chat platform, AnonAI provides users with a secure and versatile experience, eliminating the need for account logins or tracking. Moreover, for those seeking safest chat rooms or an effective AI chat tool, AnonAI sets a benchmark in secure and user-friendly AI interaction.
4. Challenges in Vision-Language Models
While VLMs are transformative, they face several challenges:
- Data Quality and Bias: Poor or biased training data can lead to inaccurate or unfair outputs.
- Computational Complexity: VLMs require significant computational resources for training and deployment, limiting accessibility for smaller organizations.
- Contextual and Abstract Understanding: While VLMs excel at specific tasks, their ability to handle abstract or nuanced inputs remains limited.
- Ethical Concerns: The misuse of vision-language models for generating misleading or harmful content poses risks that need to be addressed.
5. The Future of Vision-Language Models
The potential of vision-language models continues to grow as advancements in AI push the boundaries of what’s possible. These systems are expected to:
- Collaborate in multi-agent frameworks, much like the advanced AI agents seen today, to solve complex, dynamic problems.
- Integrate seamlessly into daily life, offering personalized assistance and creativity-enhancing tools, as demonstrated by innovations in autonomous AI agents.
- Expand into industries such as education, law, and entertainment, driving innovation and accessibility.
New vision-language models like the Qwen model and its advanced iterations, Qwen 2.5 and Qwen2 VL, are paving the way for next-generation AI systems capable of more nuanced multimodal reasoning and applications.
As vision-language models evolve, their ability to combine visual and textual reasoning will revolutionize industries, improve accessibility, and create new possibilities for human-AI collaboration.
6. FAQs
What is a large vision-language model?
A large vision-language model refers to a VLM that has been trained on massive datasets containing millions of image-text pairs. These models, such as CLIP and Flamingo, are capable of performing complex multimodal tasks across diverse domains with high accuracy.
Are there open-source vision-language models?
Yes, several open-source vision-language models are available, such as:
- Llama 3.2 Vision: Combines visual and textual reasoning in an open-source framework.
- BLIP (Bootstrapping Language-Image Pretraining): Offers robust capabilities in captioning and visual question answering.
These models provide accessibility for developers and researchers to experiment and deploy VLMs.
Conclusion
Vision-language models are shaping the future of AI by combining the strengths of computer vision and natural language processing to deliver powerful multimodal capabilities. From improving healthcare diagnostics to enhancing creative workflows, these systems are opening up new possibilities across industries.
Platforms like AnonAI, which leverage VLM technologies like Llama 3.2 Vision, exemplify how AI can be used to provide innovative, privacy-focused solutions. As these technologies continue to advance, they will redefine human-AI interaction and drive innovation across diverse fields.
Get exclusive rewards
for your first Autonomous blog subscription.
Spread the word
You May Also Like